Anthony Cocciolo
Dean of the School of Information
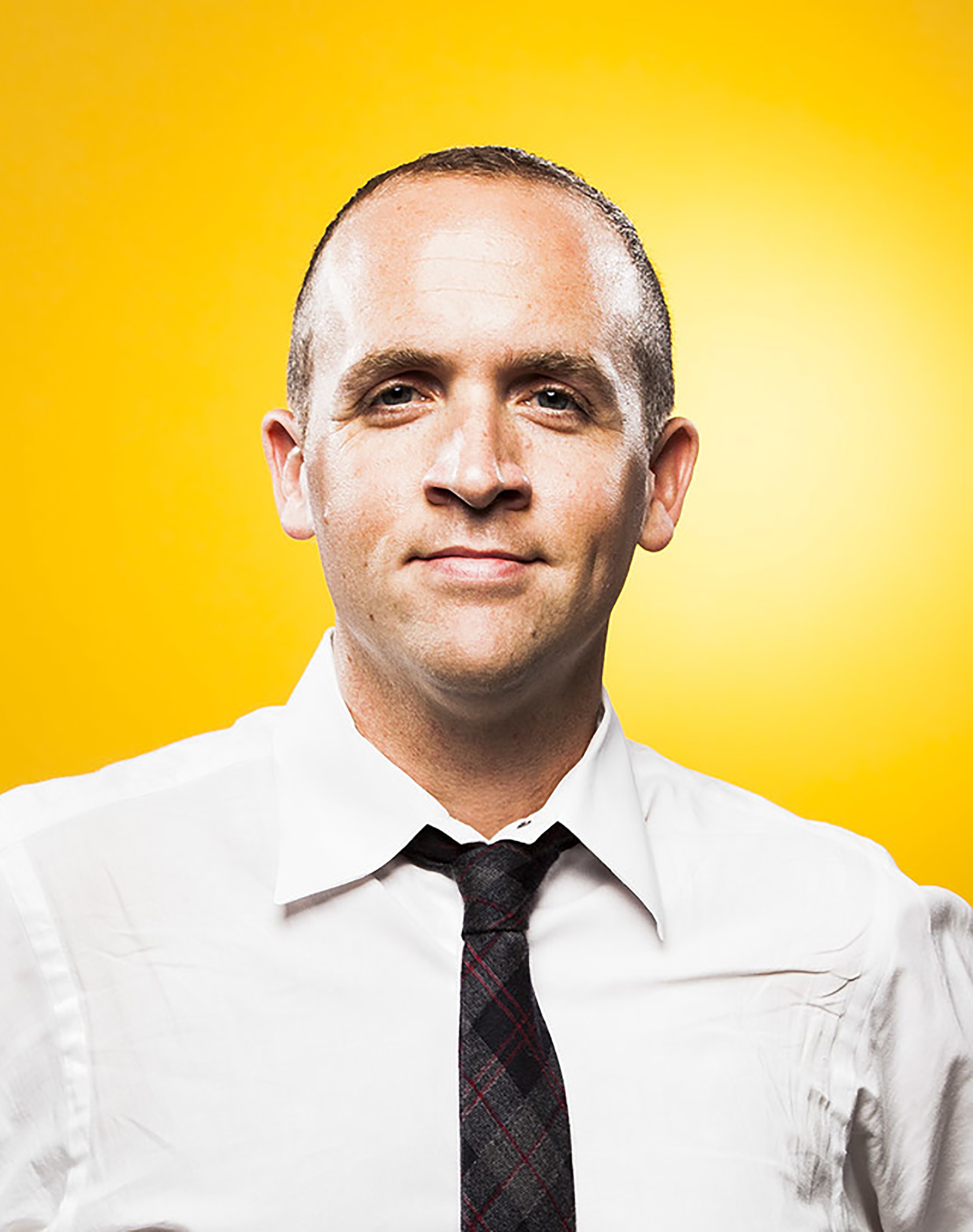
Biography
Anthony Cocciolo is the Dean of the School of Information and has been a member of the faculty since 2009. His research and teaching are in the area of archives and digital preservation, and he is the author of Moving Image and Sound Collections for Archivists (Chicago: Society of American Archivists, 2017). He is currently the Principal Investigator for the Digital Preservation Outreach and Education Network (DPOE-N) project funded by the Mellon Foundation.
Education
B.S. Computer Science, University of California at Riverside
Ed.D., Ed.M., M.A. Communication, Media, and Learning Technologies Design, Teachers College, Columbia University.